英国莱斯特essay代写 推特模型预测
Keywords:英国莱斯特essay代写 推特模型预测
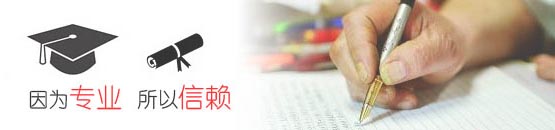
在进一步的发展中,人们提出了基于tweets的足球比赛结果预测模型(Stylianos Kampakis,Andreas Adamides, University College London.2014),并研究了这些模型是否能够成功地预测使用历史数据和统计数据的预测模型。第三个模型是用历史数据和Twitter数据构建的。最后一个模型由Cohen’s kappa(它是一种统计方法,用于计算两个评分者,当每个人对同一样本的一个试验进行评分时)测量,结果显示基于twitter的模型比使用历史数据和简单统计的模型表现得更好。如果我们将这两个模型结合起来,我们可以获得比单个模型更高的性能。因此,twitter数据可以为预测足球比赛提供有用的信息。使用的数据集有twitter数据集、历史数据集和组合数据集。twitter的数据集是使用twitters的流API创建的,该API包含200万条他们最喜欢的俱乐部粉丝的tweet。已经创建了与每个团队相关的hashtags列表。带有多个团队的hashtag的tweet已被丢弃,如果特定团队中有多个hashtag,则将其分配给该团队。使用TwitterNLP处理数据。比赛结果以主队获胜、客队获胜或平局来衡量。每个匹配被视为一个实例,它有三个特性:home、away和一个响应变量。输入包括本地功能和外部功能。对于twitter模型,主队和客队使用不同的词包作为输入数据集,使用卡方进行预处理。例如,阿森纳和曼城将拥有与主场球队相同的词汇量,但两者作为客场球队将有不同的特点。采用朴素贝叶斯模型、随机森林模型、支持向量机模型和逻辑回归模型。研究发现,随机森林是twitter模型的最佳分类器。精度高于朴素贝叶斯的精度,但对于历史数据集,朴素贝叶斯是最好的。在组合模型中,随机森林是最好的分类器,在使用bigram时,其性能最好。因此,我们可以得出结论,Twitter包含的信息足以预测一场足球比赛的结果。
英国莱斯特essay代写 推特模型预测
In further development, models for predicting the results of football matches based on tweets (Stylianos Kampakis,Andreas Adamides, University College London.2014) has been proposed and researches were made whether these models can succeed over the predictive models which use historical data and statistics. A third models was constructed with both historical and Twitter data. The final model when measured by Cohen’s kappa (It is a statistic measure used to calculate two raters when each individual rate one trial on the same sample) revealed that twitter-based model performed more better than the model that using historical data and simple statistics. And if we combine both the model, we can achieve a performance higher than that of individual models. So, twitter data can provide useful information for the prediction of football matches. The datasets used were twitter dataset, historical dataset and combined dataset. The twitter dataset was created using twitters’ streaming API which consists of 2 million tweets of fans of their favourite clubs. A list of hashtags associated with each team has been created. The tweets, that have hashtags for more than one team has been discarded and also if it there is more than one hashtag on a particular team it was assigned to that team. TwitterNLP was used to process data. The results were measured as a win for the home team, a win for the away team or a draw. Each match, which was considered as an instance, has three features home, away and a response variable. The input consists of home features and away features. For twitter model different bag of words has been used for home team and away team as input dataset, which was pre-processed using chi-square. For example, Arsenal and Manchester City will have the same bag-of-words as home team, but both will have different features as way team. Naïve Bayes, Random forests, SVM and Logistic regression models were used. It was found that, the random forest was the best classifier for the twitter model. The accuracy was higher than the Naïve Bayes accuracy achieved, but for the historical dataset, Naïve Bayes was the best. Random forest was the best classifier in case of combined model, and the best performance of it was achieved when using bigrams. So, we can conclude that Twitter contains information which is enough to predict the results of a football game.
本段内容来自网络 并不是我们的写手作品 请勿直接剽窃,查重100%,造成后果与本站无关。如需定制论文请记得联系我们。